
🚀 Dear Ark friends,
🧐Lately, ChatGPT is a hot topic in every conversation. However, this is not the only AI technique being explored in the healthcare sector. Here we tell you about 5 techniques of AI that are also breaking it in the sector.
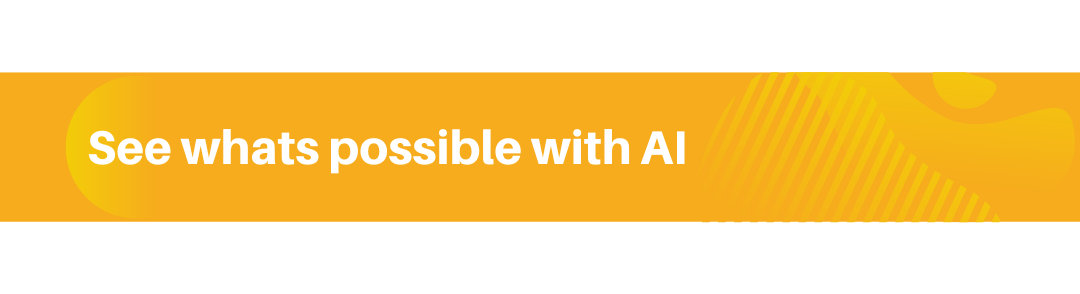
The ante-room of artificial intelligence
The term "artificial intelligence" can be misleading because due to the overuse of the expression, its meaning began to be inflated. It implies software with cognition and sentience, a much more developed technology than is used most of the time. A growing number of algorithms promise to record and transcribe meetings/conversations automatically, analyze the content, and provide you with a searchable summary. Some of these solutions are designed specifically for medical use, others are aimed at a more general audience.

Pattern recognizers rule the world
Machine learning is the field of computer science that allows computers to learn without being explicitly programmed based on computational statistics and data mining. As with traditional statistics, machine learning requires sufficient training data sets and the correct algorithms to optimize their performance on the training data set prior to testing.
Supervised learning is typically used for classification problems. You have input and output data, and the algorithm learns rules of thumb about how to categorize. It is the most popular type of machine learning in medicine and, in a few years, will be widely used in medical imaging in radiology, pathology, and other image-intensive fields.
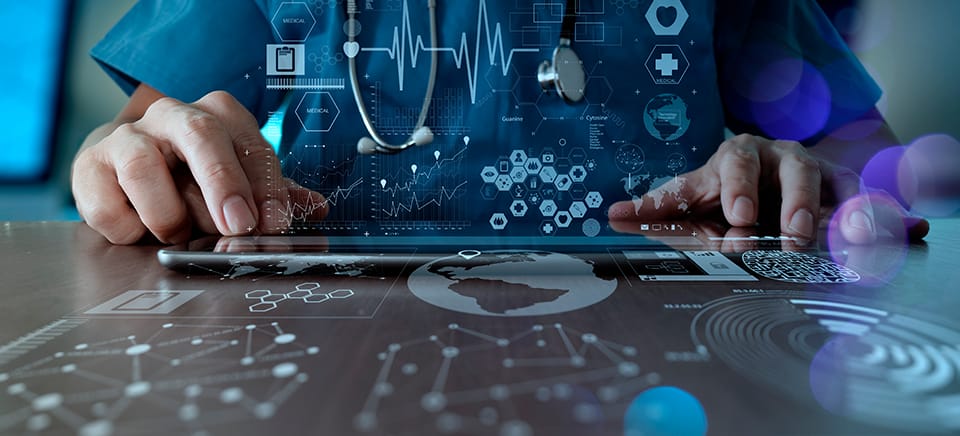
Supervised machine learning can also be effectively implemented to predict health events based on various input data, which can be classified in a linear fashion. For example, from statistics on measles vaccination rates and disease outbreaks from the Centers for Disease Control and Prevention, as well as non-traditional health data, including syndromic surveillance and social media data generated by Using software that pulls from a wide variety of medical record sources, an algorithm can provide a reliable map of future measles outbreak foci.
Unsupervised machine learning and drug-drug interactions
In the case of unsupervised machine learning, the computer program is asked to discover inherent structures and patterns found within the data. Unlike in the case of supervised machine learning, the data sets are not labeled or structured. It is generally used to predict unknown outcomes and determine how to discover hidden patterns. In medicine, for example, tissue samples can be grouped according to similar gene expression values using unsupervised learning techniques. As an example of association rule learning algorithms, one can mention the testing of new drug interactions.

Deep learning in medicine
Deep learning is the subfield of machine learning in which computers learn with the help of layered neural networks. Deep learning algorithms generally deal with messy data sets and piles of unstructured information to try to provide answers to difficult questions.
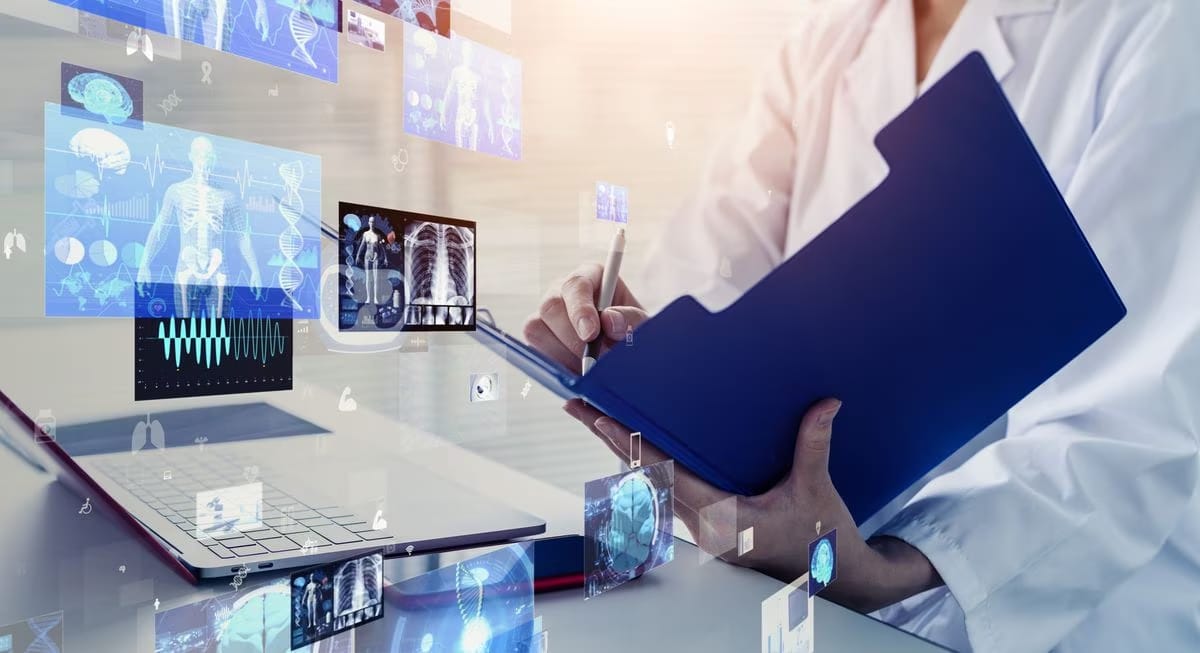
As an example of deep learning in medicine, the researchers proposed an approach to deduce treatment policies for septic patients by using continuous state space models and deep reinforcement learning. In another study, experts attempt to solve the difficult problem of estimating the size of polyps using colonoscopy images or videos, which is crucial for making a diagnosis in colon cancer screening.
The new AI medical chatbots
Just a few weeks after the launch of ChatGPT, Google/DeepMind announced the launch of MedPaLM, a large language model designed specifically to answer healthcare-related questions based on its 540 billion parameter PaLM model. Such a chatbot provides a number of potential medical applications, including knowledge retrieval, clinical decision support, summarizing key findings in studies, and triaging patients' primary care concerns, among others.

Let’s talk if you have any ideas to implement generative AI in your medical workflow!
If you have AI healthcare use cases in mind, you can tell us here.
If you enjoyed this newsletter, don't forget to share it with others and/or give us
Best regards,
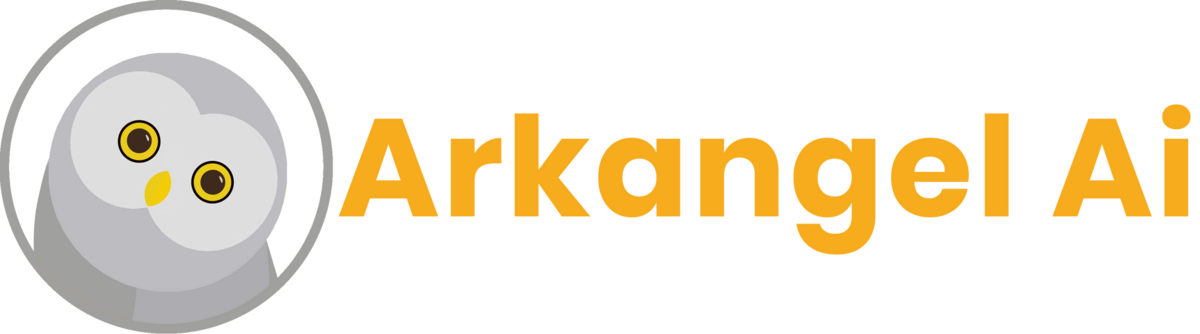